The primitives of AI
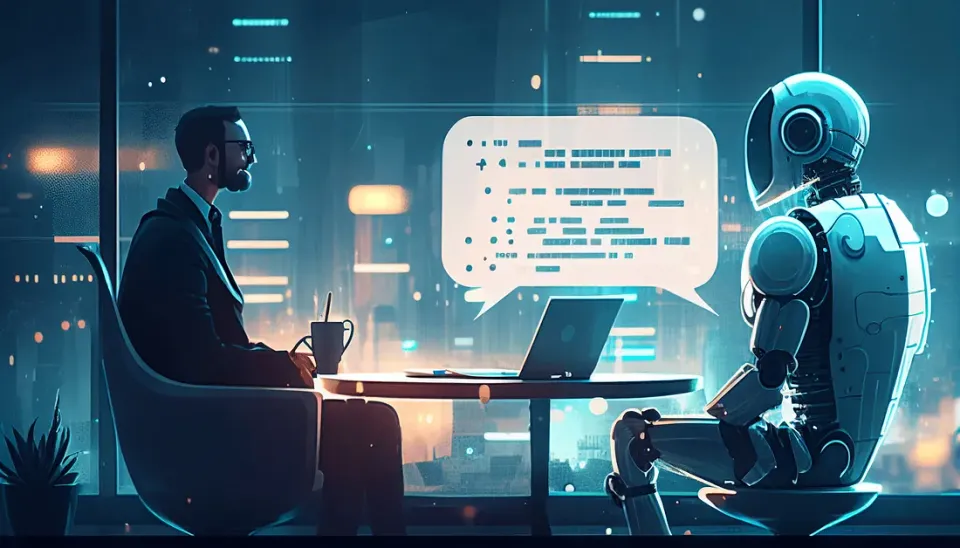
Back in 2009, Albert Wenger from Union Square Ventures wrote this piece on the opportunity in mobile. In it, he argued that the most exciting opportunities will be those that are “native” to the platform and take advantage of mobile-specific primitives. Those primitives, according to Wenger, were: location, proximity, touch, audio input, and video input.
In an effort to keep looking backward to move forward, I’ve been thinking about what the primitives for AI technology may be, to see if that might help me think through what opportunities are most exciting in AI.
Here’s a starter list that I’ve come up with:
- Generation - text, image, audio, video. Each week, it seems, we see increasingly impressive advances in content generation across the board. Sora (OpenAIs video generation model) was just the latest.
- One exciting (if a bit uncomfortable) fact about AI models is that they are eerily good at mimicking humans (so much so that I debated adding “mimicry” as a separate primitive). Anybody can experience this by prompting one of the foundational models to generate content “in the style of” a particular person. But there are countless tools out there based on leveraging mimicry alone to create novel experiences…tools that let you chat with a specific person, test a product with somebody who looks like your average user, survey someone who looks like a likely voter, and so on.
- Hallucination - hallucinations are a completely novel type of response from an AI model, and as Andy Weissman wrote here, they may indeed be a “feature, not a bug” of AI. I think of hallucination as the native ability to add randomness. The better we get at controlling hallucinations, the more we can leverage AIs hallucination capability as a “randomness dial” that we can turn up and down depending on the use case. Randomness may be useless (and even harmful) in some scenarios, but evocative and catalyzing in others.
- Retrieval & Memory - this was already getting to be true with advances in RAG, but as was made clear in the Gemini 1.5 paper released last week, AI is going to get really, really good at near-perfect information retrieval, even from a massive, multi-modal corpus, very soon. While the underlying technology will likely be different, near-perfect information retrieval looks an awful lot like near-perfect memory recall, so I’ve put these two primitives together in the same category.
- Multi-modal input - and last but not least, the latest AI models can now understand text, image, audio and video input, making them nearly as flexible in understanding as a human counterpart.
(Note: one primitive I did not mention, but I imagine is coming soon, is action. Takk a look at the “large action model” from Rabbit as an example for how a startup, at least, is approaching this problem.)
What makes this moment particularly thrilling is that these primitives, unlike during the mobile era, are themselves brand new. The mobile era was exciting because there was a convergence of existing capabilities in one device. The AI era is exciting because a whole set of new-to-this-world capabilities are converging with the capabilities we already have. I don’t know which AI native applications will emerge as winners, but I’m particularly interested in those companies that do the best job of marrying the new and the old.
As an example, we already have tools that are pretty good at answering analytical questions (e.g. coding languages). With AIs native generation and retrieval capabilities, it would seem that someone could build a 10x better research and analysis platform. If you think about how you complete research tasks today, you likely spread your work across myriad tools. Lots of searching, reading, note-taking, cross-referencing, reading again, and so on. It’s iterative, cyclical and creative. I had a professor who said good research is hard because it requires unbounded exploration while keeping your feet planted firmly on the ground. This seems like an ideal task for a model with near-perfect memory and information retrieval over one set of content and inhuman synthesis capabilities across every piece of content ever produced. The particular feature set will depend on the target customer, but this sort of platform would seem particularly valuable for industries where the upside to more/better/faster ideas is clear. Perhaps a hedge fund analyst searching for alpha?
As I’m imagining what that product might look like, I can’t help but think that by mashing together new capabilities with the old, you end up with a product that feels plucked from science fiction and almost unreal. It’s too much like a companion, not enough like a piece of software. But I think that’s the world we’re entering.
I’ll keep riffing on these ideas over the next few weeks. But would love to hear your feedback. Which primitives did I miss? What application categories are most interesting to you? And if you’re building something that’s bringing together the old and the the new…I’d love to hear about it.